A lot of business investments aim to reinforce this process in order to grant higher performance products. Data Quality directly impacts the outcome of Machine Learning algorithms and data testing has proved that good data can actually refine the ML algorithms during the development phase.
Machine Learning Artificial Intelligence And Data Quality Liliendahl On Data Quality
Supervised learning is the machine learning task of learning a function that maps an input to an output based on example input-output pairs.
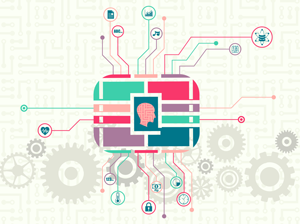
Data quality with machine learning. Garbage in garbage out. Big Data has made Machine Learning ML mainstream and just as DQ has impacted ML ML. Its vital that any machine learning engineer must have an understanding of the.
Data quality is crucial to todays enterprise you simply cant make good decisions without it. But there is a no-size-fits-all solution for a business. The quality of the predictions directly corresponds to the quality of data you train the model with.
Increased data volumes put companies under pressure to systematically manage and control their data assets. Data Quality matters for machine learning. Machine learning works so quickly that computers can perform jobs at speeds that used to be considered impossible.
As a data science architect or quality assurance QA professional dealing with quality of machine learning models you must learn some of these challenges and plan appropriate development processes to deal with these challenges. It is capable of delivering precise business insights by evaluating data for AI-based programs. In last years Machine Learning solutions play a key role in this program of investments for their ability to easily adapt in every contest and for the great results achieved.
At a high level machine learning is instrumental for data observability at scale. Efficiency considerations over suboptimal. Another important problem that machine learning can help to overcome is missing data.
Check out this article on where it makes sense to use AI and how to properly apply it. This includes checking for consistency accuracy compatibility completeness timeliness and duplicate or corrupted records. In this blog I wanted to focus on how Big Data is changing the DQ methodology.
Were going to go through all the concepts with concrete code examples. There is a close connection between Data Quality and ML tools and the long-range monetization prospects of high-quality data used in the industry. Improving Data Quality and Closing Data Gaps with Machine Learning Tobias Cagala July 14 2017 Abstract The identification and correction of measurement errors often involves labour intensive case-by-case evalu-ations by statisticians.
Machine learning allows you to improve data quality quickly and efficiently. In a nutshell a machine learning model consumes input data and produces predictions. Why It Matters in Machine Learning.
In my last blog I highlighted some of the Data Governance challenges in Big Data and how Data Quality DQ is a big part of Data Governance. We show how machine learning can increase the efficiency and effectiveness of these evaluations. For a retail demand forecasting system we can collect data over multiple years meaning weve got ample.
Quality Control is an important step in every production system. Role of a machine learning engineer. Achieving the data quality required for machine learning As Microsofts Krasadakis indicates assessing and improving data quality should be the first step of any machine learning project.
Using Machine Learning for Data Quality. The importance of the type and quality of the data in machine learning has been widely recognized especially where supervised learning is concerned. And to generate the high-quality training data for machine learning or AI you need a highly skilled annotators to carefully label the information like.
An application of machine learning to data quality management we demonstrate that the potential of machine learning for official statistics is not limited to the prediction of measurement errors. Why Use Machine Learning to Improve Data Quality. Detectors outfitted with machine learning can apply more flexibly to larger numbers of tables eliminating the need for manual checks and rules as discussed in Parts I and II as your data warehouse grows.
Unsupervised machine learning is a savior when the desired quality of data is missing to reach the requirements of the business. In addition common data management practices lack sufficient scalability and do not have the capacity to manage ever-increasing data volumes. These labeled training data is useful for the ML model since then it differentiates data categories more accurately.
It infers a function from labeled training data consisting of a set of training examples.
Using Machine Learning For Data Quality Talend
How To Improve Data Quality For Machine Learning By Jack Tan Towards Data Science
How To Use Machine Learning In Data Quality Testing Machine Learning Artificial Intelligence Data Annotation
Artificial Intelligence And Data Quality
Garbage In Garbage Out Automated Machine Learning Begins With Quality Data By Odsc Open Data Science Medium
Impact Of Data Quality On Machine Learning Results Cas
9 Data Quality Issues That Can Sideline Ai Projects
How To Improve Data Quality For Machine Learning By Jack Tan Towards Data Science
Data Quality Check In Machine Learning Youtube
Journey From Data Quality To Applied Machine Learning
Ai Enabled Data Quality For Data Quality Across Enterprise
Finding Machine Learning Ready Data Refinitiv Perspectives
The Impact Of Data Quality In The Machine Learning Era Dataversity
The Essential Guide To Quality Training Data For Machine Learning
No comments:
Post a Comment
Note: Only a member of this blog may post a comment.